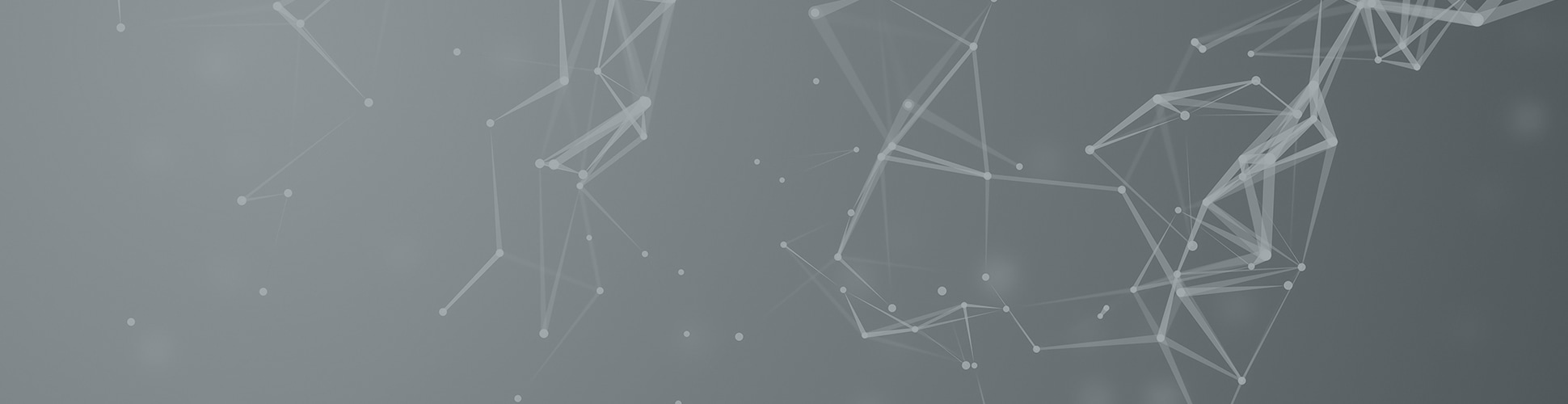
Applying Artificial Intelligence to Transform the Patient Enrollment Paradigm in Clinical Research
Learn how AI can be used throughout the drug development process, with a specific focus on patient enrollment.

Artificial Intelligence (AI) imitates human cognitive functions using computer science, algorithms, machine learning and data sciences. Simply put, AI refers to systems having the capability to perceive their surroundings, make logical deductions, interact with humans and acquire knowledge. These systems can be utilized for tasks resembling human abilities, such as recognizing images, understanding spoken words, planning and organizing.
In today’s data-driven world, AI has become valuable and indispensable, enabling organizations to extract valuable insights from vast amounts of data, make informed decisions and drive innovation across different sectors — including drug development.
The U.S. Food and Drug Administration (FDA) acknowledges the potential of AI to enhance the process of drug development in many ways, including:
- Accelerating the delivery of safe and effective drugs to patients
- Improving access to medications to promote health equity
- Enhancing manufacturing standards for better quality control
- Ensuring drug safety
- Facilitating the development of innovative drugs and personalized treatment approaches
Drug developers are increasingly integrating AI into human clinical trials to evaluate the safety and efficacy of a drug. AI can analyze large volumes of data from interventional and non-interventional studies to draw conclusions about a drug’s safety and effectiveness quicker than humans. AI also has the potential to incorporate real-world data (RWD) obtained from electronic health records (EHRs), medical claims or other data sources to inform the design and optimization strategy of clinical trials.
This blog presents examples that demonstrate how AI can be used throughout the drug development process, with a specific focus on patient enrollment.
The benefits of utilizing AI in clinical research
Patient recruitment and diversity
AI is increasingly being utilized in the recruitment process for clinical trials, aiming to connect individuals with potential benefits from investigational treatments.
For sponsors or clinical research organizations managing a clinical study, AI can be used to analyze extensive datasets, including clinical trial databases, trial announcements, social media, medical literature, registries, and structured and unstructured data in EHRs. The algorithms are trained on large volumes of patient data and enrollment criteria from previous trials to match individuals with suitable trials. For participants, AI offers the benefits of improving access to relevant trial information by enabling tools, such as AI chatbots, voice assistance and intelligent search.
With the growing importance of improving patient diversity in clinical trials to address disparities in access to safe and effective treatments, AI algorithms can be trained on diverse patient populations to ensure equitable inclusion, considering factors such as gender, race and ethnicity. Validation of the AI algorithms to ensure that the recruitment process achieves fair representation of the general population will be key to filling knowledge gaps about how treatments affect different groups, potentially resulting in disparities in health care outcomes.
Strategic selection of patients in clinical trials
AI has been explored and used to predict individual participants’ clinical outcomes based on various baseline characteristics, such as demographic information, clinical data, vital signs, labs, medical imaging data and genomic data.
Predicting clinical outcomes helps identify participants who would likely respond better to treatment and who might be at a higher risk. AI can also predict the likelihood of a serious adverse event before administering the investigational product at an individual level, allowing patients to be categorized by their risk profile. A high-risk participant can be even excluded from the study based on the severity of the adverse event.
Such intelligent selection of participants in clinical trials may also help reduce variability in outcomes and increase the statistical power of the study.
Dose/dosing regimen optimization
Selecting an appropriate dose or dosing regimen when there is limited data available on the drug in human models can be challenging for new investigational products. There’s an added level of complexity when clinical trials need to enroll specialist patient populations, like rare diseases, pediatric patients or pregnant women, where doses need to be carefully selected to keep the subject out of harm’s way.
AI has the capability to analyze and forecast the pharmacokinetic (PK) profiles of drugs following their administration. Additionally, AI can examine the correlation between drug exposure and response, including the effect of variables, thus ensuring optimal dose selection and dosing regimens for clinical research.
Patient compliance and retention
Once a subject is enrolled in a clinical trial, the subsequent challenge is to keep the patient compliant to the study protocol and avoid dropouts from trials, which cause costly delays.
AI-based smartphone alerts, reminders, electronic tracking of medication through smart pillboxes and visual confirmation tools, and e-tracking of missed clinical visits are all examples that would trigger alerts for non-compliance.
AI can also create patient profiles from data obtained from digital health technologies (DHTs) and other systems, potentially enabling the prediction of dropouts and adverse events to ensure better patient retention.
Selecting the right sites for any study
Successful patient enrollment is a byproduct of selecting the right sites for any study. AI can be employed to evaluate site performance and assess the likelihood of delays based on data from previous trials conducted at those sites. It can also help with site training and identifying process gaps to improve performance. This optimization of trial operational conduct streamlines the site selection and mitigates potential risks associated with delays.
Choose a development partner that recognizes the potential of AI in transforming the drug development process
The PPD clinical research business of Thermo Fisher Scientific’s significant investment in AI reinforces our keen focus to deliver efficient solutions to our drug development partners. Our key initiatives include the integration of AI technologies into our processes, enabling faster and more accurate data analysis.
With AI, we provide our clients with more precise insights and recommendations for strategic decision-making throughout the drug development life cycle.
We are currently applying AI in the following ways:
Clinical trial optimization: AI is employed to optimize various aspects of clinical trials, including patient recruitment, trial design and data analysis. By analyzing large datasets and applying machine learning algorithms, our teams quickly identify potential patient populations, develop optimized trial protocols, and improve the efficiency of data collection and analysis.
Predictive analytics: AI-powered predictive analytics identify potential risks and opportunities in clinical trials. By analyzing historical and real-time data, we effectively predict patient enrollment rates, anticipate potential safety concerns, and optimize trial timelines and resource allocation.
Real-world data analysis: Natural language processing and text mining extract insights from unstructured RWD sources, such as medical literature and electronic health records. This enables our experts to generate evidence-based insights and accelerate the identification of potential new drug targets or safety signals.
Virtual trials and remote monitoring: AI and digital technologies are being leveraged to facilitate virtual clinical trials and remote patient monitoring. Through wearable devices, mobile apps and AI-powered algorithms, we collect real-time patient data and monitor patient safety remotely, reducing the burden on patients and improving overall trial efficiency.