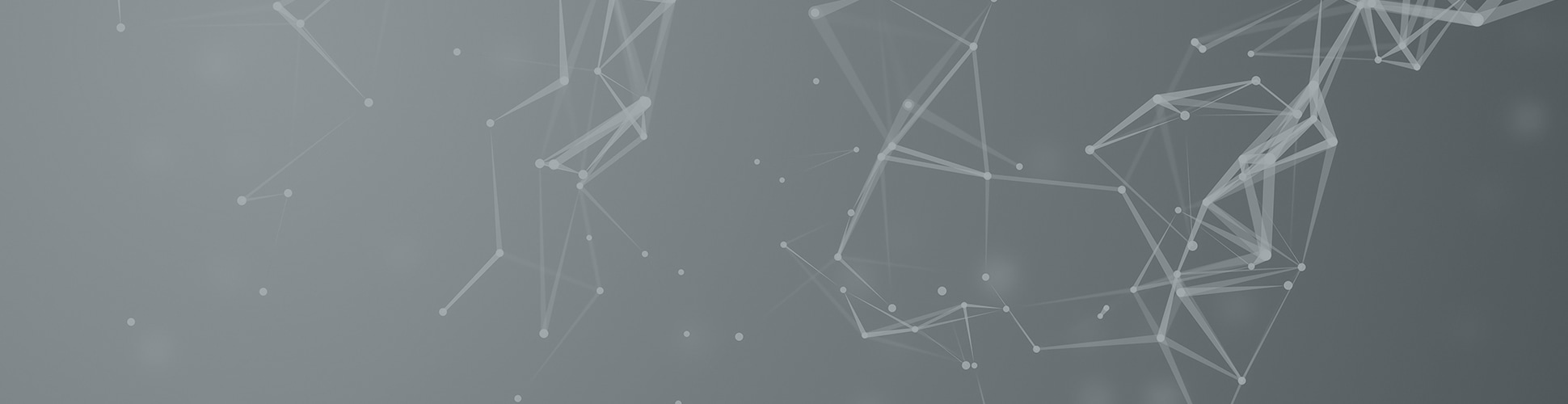
Real-World Data’s Role in Solving for Diversity in Clinical Research
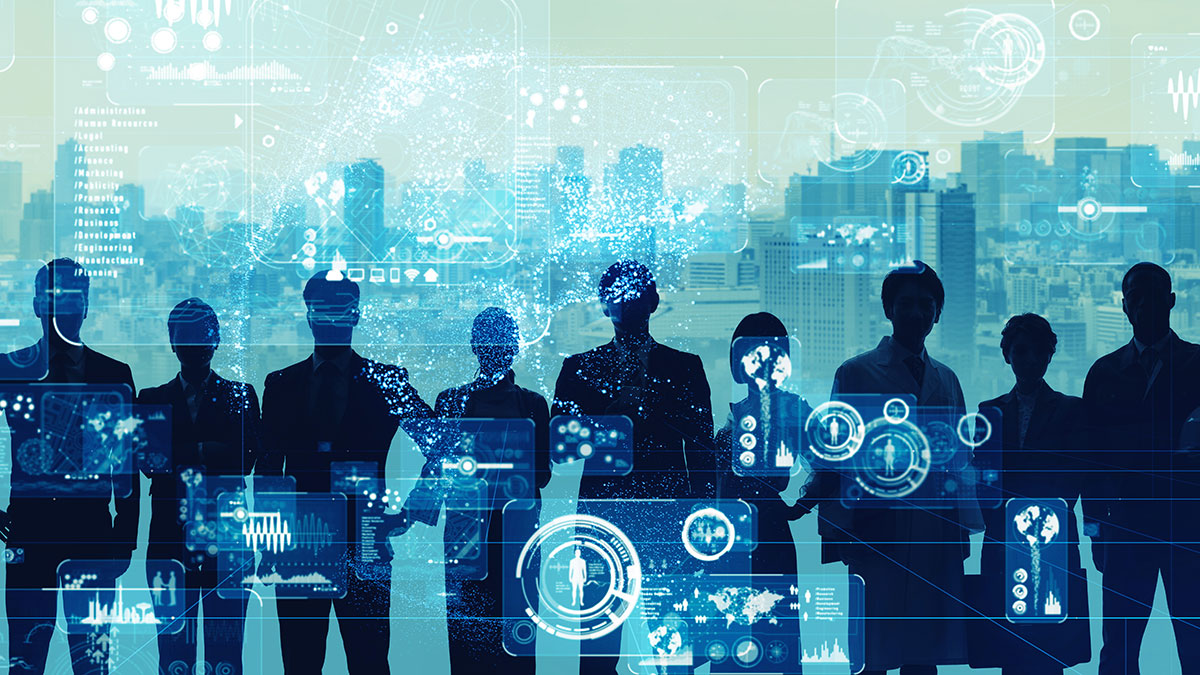
A key limitation of traditional clinical trials needed for registration is the lack of their generalizability. This issue has also taken a social dimension of diversity, but — scientifically speaking — the larger issue is of representativeness, as trials populations can never truly be representative of the general population. Diversity has become a key priority, and efforts are being made to enroll more diverse patient populations. However, true representativeness will remain a challenge, as clinical research requires stringent inclusion and exclusion criteria to meet regulatory approval, which ultimately limits representation.
In this blog post, Avanish Mishra, Ph.D., global vice president of growth initiatives, discusses how using real-world data (RWD) and real-world evidence (RWE) to supplement traditional trials provides greater understanding of safety and efficacy and effective off-label use, as well as allows for fine-tuning of dosage and other prescribing activity for specific subsets of patients.
Why true representation in clinical trials is key
In any type of research that involves the establishment of conclusions based on the assessment of a small subgroup or sample, for those conclusions to be comprehensively valid, the subgroup or sample must be as representative of the entire affected population as possible, whether that involves people or machines or other types of study items.
It has been shown that pharmacological interventions lead to different responses and have different results in different populations. These results can be attributed in part to genetics (e.g., African Americans, Asians and Caucasians all respond differently to certain drugs), environmental aspects (e.g., living at high altitude), lifestyle (e.g., diet, exercise, stress levels, health care attitudes) and culture. Different phenotypes clearly play a role in how the human body responds to medical treatments. Similarly, comorbidities and the use of other medications have the potential to impact the performance of any drug. In Japan, for example, which requires data to be generated locally on its population for approval, drugs are available in lower doses than those available in the Western world.1,2
Accounting for these various known factors — or the lack of doing so — directly impacts the knowledge that can be obtained regarding the effects of medicines and ultimately their safety and efficacy when taken by patients in real-world settings.
Real-world representation still needed
Traditional randomized clinical trials (RCTs) have strict participation criteria and often exclude patients with comorbidities, which precludes studies on the vast majority of people who are likely to take new drugs. They are also performed in highly controlled environments that do not really represent the real world. While there has been a lot of emphasis on trial site selection to capture more representative populations, it remains a challenge, as investment is needed to develop new clinical trial sites.
For these reasons, diversity has gone beyond being a sociopolitical issue to becoming a scientific one as well, because we do not have representative populations in clinical trials. It is more than a question of certain minorities not being represented. We are not able to truly understand how new drugs are widely used in the real world because we are not able to study them within a representative microcosm that addresses lifestyle, environmental and genetic differences.
Regulatory agencies have recognized the challenges created by the lack of proper representation in clinical trials. The U.S. Food and Drug Administration (FDA), for instance, issued final guidance in November 2020 about ways trial sponsors address the issue, such as through broadening enrollment criteria and avoiding unnecessarily excluding participants.
Real-world data are crucial for compensating for the limitations of clinical trial representation
Even if trials become more representative, it will never be practical to execute a true global clinical study that includes every possible type of patient and all lifestyle, environmental and genetic variations. It simply isn’t possible to study all phenotypes in a registrational trial as the costs and logistics would be too high and complex. For trials that add a few additional patients, the results will typically not be statistically significant.
It will always be essential to conduct clean, controlled trials to get as close to causality as possible to evaluate the efficacy of the drug. The key is to supplement the results from those trials with RWD. Indeed, documentation of RWD helps to further de-risk and customize treatments for different subpopulations.
RWD can be generated and collected after the new drug or medical device has been launched on the market from patients receiving treatment in a real-world setting. There is no limitation on patient numbers and no criteria that can exclude participation. Most importantly, RWD and RWE — the clinical evidence derived from analysis of RWD — include information that cannot be obtained in clinical trials.
Both the FDA and the European Medicines Agency have published guidance and frameworks for the use of RWD and RWE. They also both recognize the value of RWE in supporting the use of approved drugs in new indications and for confirming the safety and efficacy of marketed products, and they are working with various stakeholders to determine the best approaches that will maximize the value of RWE at all stages of the drug development cycle.
The issue of RWD collection
One of the greatest challenges to realizing the full representative value of RWE is a collection of relevant RWD in a systematic and unbiased manner. Fortunately, many technologies have been developed that allow for sharing and aggregation of various types of medical data, but there is work to be done to integrate those technologies and to overcome country-specific regulations regarding data usage.
Biases are less likely to exist in RWD collected by countries with national, single-payer health care systems, but they can be an issue in other countries, including the United States. The fact that RWD is most often generated during medical interventions is another issue. People who lack access to health care or choose not to use the established health care system will not have their data captured. In addition, the data only pertain to patients who are already sick, not healthy people who are taking preventive measures or are less predisposed for any reason. In the real world, we are still overwhelmingly only collecting morbid data.
One of the biggest challenges to RWD collection is the fact that the majority of relevant health care data is generated outside of the health care dimension. One approach to this problem is to collect data from apps on smartphones or wearable devices. Unfortunately, these data will still be biased, because the people who use these apps have passed a certain economic threshold and tend not to be representative of the overall population. Patient-generated data outside of health care interventions remain the “Holy Grail” and a big challenge. It is worth noting that many drug development programs are beginning to collect some of these data to inform secondary endpoints in clinical trials, which is ideal, because protocol-driven data collection like this will inevitably be cleaner than RWD.
Many sources of RWD
Sources of RWD include electronic medical records (EMRs), product and disease registries, medical claims and billing data, and patient-reported outcomes (PROs). These data can be collected as part of routine participation in the health care system and used with the help of tokenization and tokenized platforms to build a more complete picture of that patient.
The data can also be analyzed as part of different RWE studies, including comparative effectiveness studies and subgroup analytics. The former provides information on the impacts of multiple drugs for the same indication to see how each performs among different subpopulations of patients. The latter focuses on specific patient subgroups and delves more deeply into how a drug impacts that group, providing greater knowledge about safety and efficacy.
Global application opportunities
There are two important global implications for the growing use of RWD and RWE. The first is the ability to improve treatment protocols for patients in countries that rely heavily on the EMA and the FDA for their approvals. RWD gathered on local patients can be used to supplement clinical trial data conducted elsewhere on populations that have different genetic traits and lifestyles and that potentially live in very different environments. Countries such as Brazil, India and Russia should seek to identify non-burdensome mechanisms for using RWD and RWE to make label changes for their specific countries.
RWD can also be leveraged to increase the diversity of recruited patients. Analysis of RWD can provide information on trial site locations where diversity is higher. Combined with decentralized trials, RWD/RWE can dramatically improve the representativeness of patient populations. With greater numbers of clinical sites and microsites and the movement of clinical studies into the community setting, it is possible to include many more and different patients in clinical trials that would not have participated in the past.
The beginning of convergence
The path forward is fairly clear, but it will require real effort. Issues related to data capture, standardization and globalization must be addressed. Regulations are needed to ensure the right balance between patient privacy and the use of RWD for advancing medicine. There must be secure and standardized means for aggregating data and linking them on a global level, which is the ultimate goal.
The first steps have been taken, and the macro trends of diversity/representativeness, technology advances and greater acceptance of RWD/RWE are converging to advance this field. The devastation wrought by COVID-19 in countries with poor health care infrastructure will hopefully also lead to the establishment of better health care systems that will further increase the quantity of data capture.
Leading to localization and personalization of medicine
This convergence is supporting the trend in the pharmaceutical and health care sectors toward personalized and precision medicine. With more drugs designed to address the specific medical needs of individuals, conducting clinical trials becomes even more challenging. It will be too burdensome for the pharmaceutical industry to conduct clinical trials in every country and for patients with multiple phenotypes. RWD will be essential to fine-tuning the profile of drugs for their various applications.
Overall, we believe that the combination of traditional RCTs with RWD/RWE is taking the industry to a better place and making it possible to better serve patients in need. For instance, in China, it is possible to get a new drug approved in certain cases of unmet need based on a global registration package, if there is no drug available in the country for the condition. Such approvals come with a requirement for post-marketing studies to ascertain the safety and efficacy of the drug in the Chinese population.
This approach will be common in the future. RCTs will be performed to establish initial safety and efficacy, with RWD collected for RWE studies that investigate the safety and efficacy in specific patient populations. Hopefully not too far in the future, it will be cost-effective for every single person to have gene sequencing completed and their data captured and analyzed to allow for very precise tailoring of medicines.
The biopharma industry is at the cusp of mainstreaming precision medicine that leverages artificial intelligence and machine learning, and it behooves us to ensure that the data used for these algorithms are truly representative of the diversity in the general population by using RWD. Ultimately, the use of RWD will bring down the cost of drug development while providing greater representativeness, leading to improved clinical research that generates better, more complete data even more efficiently.